7 Discussion
7.1 Introduction
This research starts with the aim of developing, demonstrating, and validating an integrated data-driven approach to assisting computational urban design processes. Five objectives are set up for achieving the aim.
Understanding current data-driven urban design processes in practice and their enabling computational smart technologies;
Developing a framework to systematise an approach for data-driven urban design processes;
Developing a computational design toolkit prototype to assist urban design processes;
Demonstrating the computational toolkit prototype through a scenario;
Validating the integrated data-driven urban design approach amongst designers.
As introduced in Chapter 3, the Design Science Research methodology was applied in this research for the development of an integrated data-driven approach to assisting computational urban design processes. The development process was divided into three phases: the Conceptual Development Phase, Computational Development Phase, and Validation Phase. Through the implementation of these phases, the aim and objectives have been achieved.
The purpose of the Conceptual Development Phase consisted of creating an understanding of current data-driven urban design processes in practice alongside the enabling computational smart technologies, thereby developing an approach for data-driven urban design processes. This phase accessed Objectives 1 and 2.
From the Literature Review, it was identified that there is a lack of integrative approaches between the design process and data procedures and the need for a more holistic understanding of the use and development of data-driven urban design tools. Furthermore, the narrative review conducted supported the creation of an integrated understanding of concepts and theories of urban design, enabling computational smart technologies, and data procedures (Objective 1).
From the Semi-Structured Interviews, the previous understanding developed during the Literature Review was refined with perspectives from traditional and data-driven designers regarding current (data-driven) urban design practices and their enabling computational smart technologies (Objective 1). The conducted Thematic Analysis followed a deductive approach to identify, analyse, and connect themes that guided the development of the conceptual framework (Objective 2).
The purpose of the Computational Development Phase consisted of creating a computational toolkit prototype to assist urban design processes, which was then and demonstrated through a scenario to ensure the feasibility of the developed approach. This phase accessed Objectives 3 and 4.
From the Computational Prototyping, a computational toolkit prototype was developed to assist data-driven urban design processes. The prototype sought to access the feasibility and validity of the approach. The computational toolkit prototype was developed as a plugin for Sverchok, a visual programming environment of Blender 3D, in a context of reflective practice consisting of feedback loops of where concepts were proposed, built, tested, and retested (Objective 3). The developed tools were demonstrated in a real-world scenario to show their potential usability (Objective 4).
The purpose of the Validation Phase was to confirm the usefulness of each element of the approach and their likely use by real-world urban designers. This phase accessed Objective 5.
From the Focus Group Interviews, the integrated data-driven approach to assisting computational urban design processes, consisting of the framework and computational toolkit, was validated. The focus group data was qualitatively analysed using constant comparison between the two groups of participants (Traditional and Data-Driven Designers). The outcomes were positively validated through the three validation foci: a) systematisation of Data-Driven Urban Design Processes, b) Integration of Datasets, Design, and Data Tools, and c) Holistic Toolmaking Development via a Computational Toolkit, as well as evaluation of the potential adoption of the approach (Objective 5).
The following sections of this chapter discusses the key findings described in Chapters 4, 5, 6 providing explanations and evidence to address the five research objectives outlined in Chapter 1. This chapter is comprised of five sections. Section 7.1 introduces the chapter. Section 7.2 discusses the implications of systematisation of data-driven urban design processes. Section 7.3 discusses the role of an integrative data-design environment for data-driven urban design. Section 7.4 discusses holistic data-driven urban design toolmaking and its implications in relation to future smart cities. Section 7.5 concludes the chapter.
7.2 The Implications of Systematisation of Data-Driven Urban Design Processes
In Chapter 2, the first research gap presented in Section 2.5 highlighted that while the emergence of smart cities frameworks and smart infrastructure have enabled the exploration of data-driven urban design processes through computational design methods, there is a lack of systematic approaches integrating design processes and data procedures. This lack of systematisation was further highlighted during the analysis of the semi-structured interviews in Chapter 4, revealing that, despite the uniqueness of the design process in terms of context and designers’ background and preferences, a common structure across similar design processes could be identified to better support and guide data-driven urban design processes. The understanding developed in Chapter 2 and its further refinement, including perspectives from traditional and data-driven urban design practitioners, as presented in Chapter 4, answered Objective 1. On top of this understanding, a framework was developed combining existing frameworks and concepts presented in Chapter 2, the including cognitive steps of the design process (Gero 1990; Gero and Kannengiesser 2004; Yu, Gu, and Ostwald 2021), data procedures (Deutsch 2015; Mathers 2019; Runkler 2020; A. Liu, Wang, and Wang 2022), and computational technologies (Maguire 1991; Alonso et al. 2004; Botsch et al. 2010; Grekousis 2020; Runkler 2020; Dieck, Jung, and Loureiro 2021; Byerly, Kalganova, and Ott 2022), and the findings from Chapter 4. The development of the conceptual framework answered Objective 2. The benefits of the proposed systematic structure of data-driven urban design processes include better support for evidence-based decisions during data-driven urban design processes. These are necessary due to the increasing demand for evidence-based solutions in urban design resulting from the recent “data tsunami” (Kvan 2020). Additionally, the proposed systematisation provides guidance for urban design professionals seeking to explore data-driven processes. Furthermore, the proposed systematisation of data-driven processes can be used for the development of new data-driven tool implementations, as demonstrated by the computational toolkit prototype developed and presented in Chapter 5, contributing to Objectives 3 and 4.
During the research validation process presented in Chapter 6, both traditional designers and data-driven designers highlighted the potential for a cohesive systematic approach implemented as a computational tool in a visual programming environment, providing evidence and face validity regarding the feasibility and usability of the proposed approach and contributing to Objective 5.
From an overall perspective of the contribution to knowledge, this key finding revealed that both traditional designers and data-driven designers follow similar steps during the design process. However, there is a crucial aspect that differentiates traditional and data-driven processes in terms of the relationship between design cognitive steps and data procedures, which was discussed in Section 4.3.1. While in the traditional design process, documentation is the final step that creates a graphical narrative of the final design outcomes, the iterative nature of the data-driven process re-signifies the role of documentation as a potential re-formulator of Function-Behaviour-Structure, adding an extra layer of potential reformulations, as shown in Figure 7.1, and, consequently, potential new perspectives for further explorations of the solution space of the design process.
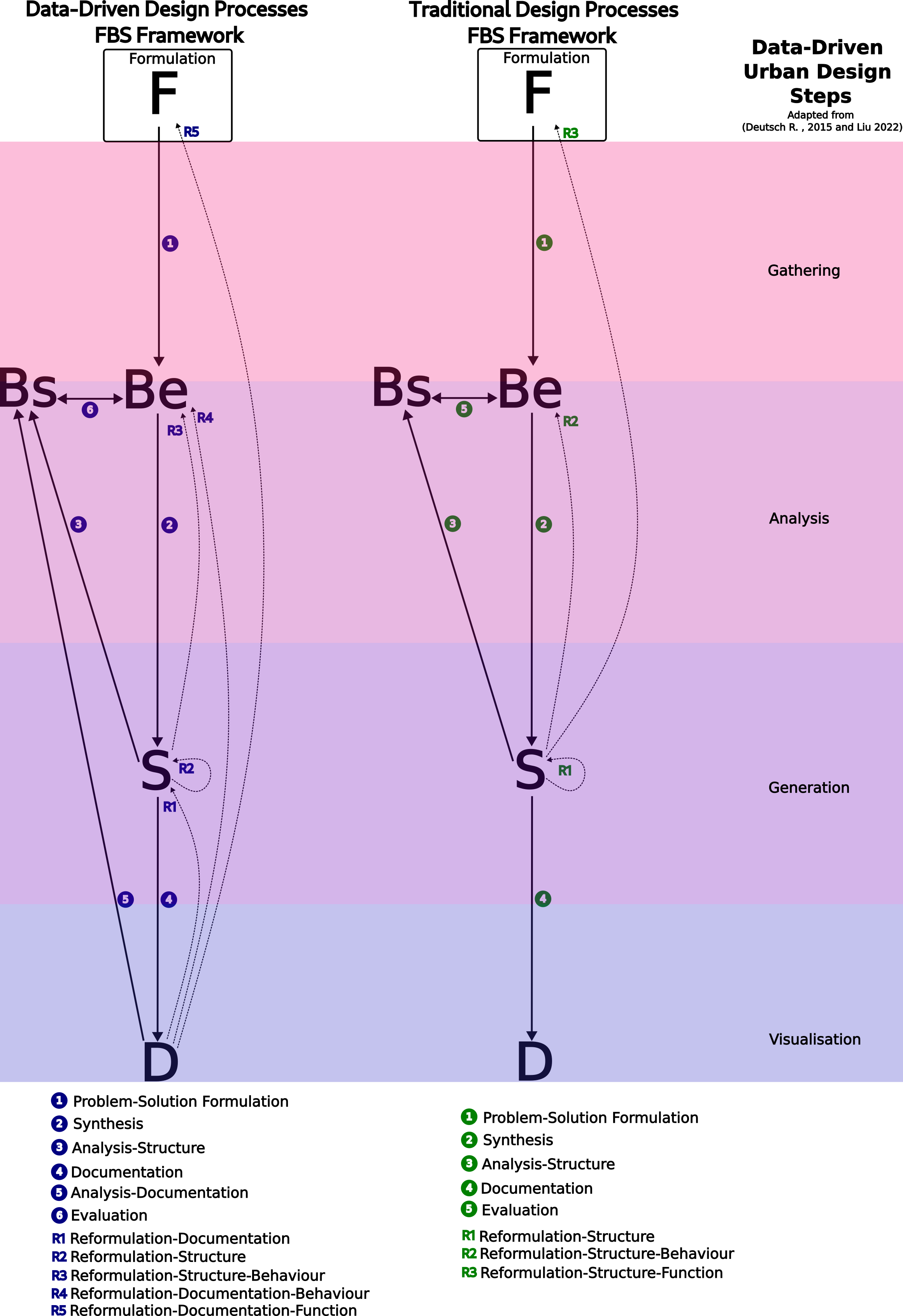
Figure 7.1: FBS Framework comparison traditional and data-driven designers adapted from Gero (1990)
This key finding is supported by qualitative evidence; however, further studies that include mixed methods of qualitative and quantitative analyses, such as protocol analysis and statistical analysis, could be conducted to further enhance understanding of the similarities and differences in cognitive design steps between traditional designers and data-driven designers. By using mixed methods that combine qualitative and quantitative analyses, researchers can gain a more comprehensive and nuanced understanding of the cognitive design steps employed by these two groups of designers.
Qualitative protocol analysis can provide rich insights into the cognitive processes and strategies employed by designers as they engage in design tasks. Protocol analysis involves the collection and analysis of verbal or written accounts of participants’ thoughts and actions during the design process, allowing for a deep exploration of their decision-making processes, problem-solving strategies, and creativity in design. For example, think-aloud protocols, where participants verbalize their thoughts as they engage in the design process, and retrospective protocols, where participants reflect on their design decisions after completing a design task, can provide valuable qualitative data that captures the cognitive design steps of traditional designers and data-driven designers (Ericsson 2006).
On the other hand, quantitative statistical analysis can provide objective and empirical insights into the cognitive design steps employed by designers. Statistical analysis can be used to analyse quantitative data, such as the time taken for each design step, frequency of revisiting design decisions, and cognitive load measures, which can provide quantitative measures of the design process. Descriptive statistics, such as mean, median, and standard deviation, can summarize and describe the data, while inferential statistics, such as t-tests or ANOVA, can be used to compare the differences between traditional designers and data-driven designers in terms of their cognitive design steps (Field 2018).
By combining qualitative protocol analysis and quantitative statistical analysis, researchers can triangulate their findings and validate the results from multiple perspectives. The qualitative data can provide rich insights into the cognitive processes and strategies employed by designers, while the quantitative data can provide objective measures of the design process. This mixed methods approach can lead to a more comprehensive understanding of the similarities and differences in cognitive design steps between traditional designers and data-driven designers and can contribute to the advancement of knowledge in the field of design. However, it should be noted that this type of analysis is beyond the scope of this research.
7.3 An Integrative Design-Data Environment for Data-Driven Urban Design
The literature reviewed in Chapter 2 explored the complexity of problem-solving in urban design processes, focusing on multiple dimensions (Carmona 2002, 2021; Boyko, Cooper, and Davey 2005; Batty 2013b) and requiring designers to combine multiple layers of information from different datasets within different software solutions. Additionally, it highlights the importance of easy access to data and interoperability for the success of an urban design project.
The complex nature of urban design processes discussed in Chapter 2 was further highlighted in the findings of Chapter 4, which revealed a diverse range of urban design themes cited (Tables 4.6, 4.4, and 4.5). Additionally, the chapter illustrates the relationship between the creation of urban design narratives and the availability of computational tools among data-driven designers (Tables 4.5 and 4.24), suggesting a demand for the exploration of overlooked urban design dimensions to support data-driven designers in creating stronger and more holistic urban design projects. The lack of interoperability of data and design tools was identified as a barrier to urban design workflows, as found in both the literature review and the interviews. This highlights the importance of integrating data and design tools to better support evidence-based and data-driven urban design processes (Deutsch 2015; Mathers 2019; Kvan 2020), by providing real-time feedback and improving interoperability of data-driven urban design processes. Understanding of the integration of design and data tools to better support data-driven urban design processes also addresses the first research gap presented in Section 2.5 and contributes to answering Objective 1 and 2.
The study found that integrating design and data tools, as presented and developed in Chapter 5, improves designers’ experience regarding the interoperability of tools and accessibility of data, enhancing the overall workflow of data-driven urban design processes, as discussed in Chapter 6 on research validation. The comprehensiveness and cohesiveness of the developed approach, which includes design and data tools covering seven distinct computational technologies and its integration into a single visual programming environment (see Chapter 5), instead of using multiple pieces of software, was highlighted as a factor that contributes to enhancing urban design workflows. This discussion around design and data tools presented in Chapters 5, and 6 contributed to answering Objectives 3, 4 and 5. However, the use of visual programming can have a steep learning curve for new users, as it involves a shift in thinking that unfolds the subjectivity of the design process towards making explicit every decision and step of the design process in algorithmic thinking fashion (Oxman 2017). This can create a barrier for experienced traditional designers used to their own subjective processes. Therefore, the developed toolkit prototype provides visualisation tools in the form of interactive dashboards (see Chapter 5) that communicate with the visual programming environment to bridge the communication gaps between traditional designers, other stakeholders and data-driven designers, serving as a common ground for seamless collaboration. The use of interactive dashboards was positively received by both traditional and data-driven designers, with traditional designers being the most enthusiastic in highlighting its potential, as shown in Chapter 6.
Although interactive dashboards can potentially enhance collaboration among different stakeholders, it is only a small step in bridging the collaboration gap between data-driven and traditional design methods. Beyond the scope of this research, further investigation into collaborative co-design platforms involving stakeholders with multidisciplinary backgrounds could advance understanding of how to bridge this gap. By investigating these platforms, insights could be gained as to how they can be used to enhance collaboration and improve the outcomes of data-driven urban design projects.
One key aspect of collaborative co-design platforms is their ability to facilitate communication and knowledge sharing among stakeholders with diverse backgrounds. These platforms provide a virtual space where stakeholders can collaborate in real-time, share ideas, provide feedback, and co-create design solutions. Through this collaborative process, stakeholders can pool their multidisciplinary expertise, perspectives, and insights, leading to more informed and holistic design decisions. This investigation can shed light on how collaborative co-design platforms can enable effective communication and knowledge sharing among stakeholders, and how these platforms can be designed to accommodate the specific needs of data-driven urban design projects.
Another aspect of collaborative co-design platforms is their potential to support participatory and inclusive design processes. Data-driven urban design projects often involve multiple stakeholders, including community members, who have different perspectives, interests, and expertise (Carmona 2002). Collaborative co-design platforms can provide a democratic and inclusive environment where all stakeholders can contribute to the design process, regardless of their disciplinary background or level of expertise. Such an investigation could explore how collaborative co-design platforms can facilitate participatory design processes, empower stakeholders with diverse backgrounds, and ensure that all voices are heard and integrated into the design outcomes.
Furthermore, investigating collaborative co-design platforms can provide insights into the role of data and technology in the collaborative urban design process. These platforms regularly leverage data and algorithms to facilitate decision-making and design exploration. This investigation can examine how the integration of data and technology in collaborative co-design platforms can enable stakeholders to access, analyse, and visualise complex urban data, and how this can inform design decisions. It can also explore the challenges and opportunities of using data and technology in the collaborative urban design process, such as issues related to data privacy, data quality, and algorithmic bias.
Overall, investigation into collaborative co-design platforms involving stakeholders of data-driven urban design with multidisciplinary backgrounds can contribute to the advancement of knowledge in the field by providing insights into how to bridge the collaboration gap among stakeholders with diverse backgrounds, promote participatory and inclusive design processes, and leverage data and technology in the collaborative urban design process (Nam and Pardo 2011; Verhoeff, Merx, and Lange 2019). However, investigating co-design platforms in relation to data-driven urban design is beyond the scope of this research.
7.4 Holistic Data-Driven Urban Design Toolmaking and Future Smart Cities
As explored in Chapter 2, due to the increasing customisation and complexity in design projects and the lack of flexibility of commercial tools in adapting to this trend, designers are becoming developers of their own tools, known as “digital toolmakers” (Ceccato 1999; Fischer, Fischer, and Universtität 2003; Burry 2011). Despite the acceleration of this toolmaking process among designers using visual programming tools, current trends show that digital toolmakers in design tend to rely exclusively on proprietary software. This trend is reinforced in the findings of Chapter 4, as shown in Tables 4.22, 4.23, and 4.24. The use of proprietary software restricts and limits user-developers’ freedoms in utilising, sharing, and collaborating through these pieces of software (Stallman 2002). However, design tools for designing cities should be understood as collective, co-created socio-political technological artefacts, empowering citizens to have the “right to the city” (Lefebvre 2008) within the context of a future smart cities framework that aims to bridge the gap between human-centric and technology-driven approaches (Kummitha and Crutzen 2017). An holistic digital toolmaking process should consider the socio-political implications of the use and development of tools for data-driven urban design. This concern is highlighted as the second research gap presented in Section 2.5. This holistic understanding of the digital toolmaking process contributes conceptually to answering Objectives 1 and 2.
Also, computationally, this holistic understanding of the digital toolmaking process, contributed to answering Objectives 3 and 4, since the developed computational toolkit prototype was created through an holistic toolmaking process, using exclusively Free and Open-Source software, from its conception to the final computational prototype. As a usable computational prototype, it is publicly available, along with its source code, on GitHub30 under a Free and Open-Source GPL-3 license. Since the computational prototype is a piece of free software, it is intended that a community of users and developers collaborate for further improvements according to data-driven urban design community demands, in the spirit of free and open-source development and an holistic view of digital toolmaking.
Although most data-driven designers use proprietary tools as their daily-drivers for the use and development of tools for urban design, as shown in Chapter 4, the findings from Chapter 6 show that they are willing to use and develop open environments, and they recognise the technical and social benefits of using free and open-source tools, such as faster updating, bug fixing, alongside public participation and community value, thereby contributing to answering Objective 5.
Although the computational toolkit prototype was completed as a research artefact, it is an ongoing, community-driven development that continues to improve and expand its functionality. Current computational toolkit implementation is limited to one specific design environment (Blender-Sverchok), written in a specific programming language (Python), and addressing a specific design subject (Data-Driven Urban Design). Later, in the light of this research, the implications of holistic digital toolmaking development could be explored beyond the urban design field, expanding its perspectives to include other areas of the Architecture, Engineering, Construction (AEC) fields, and potentially moving into other fields that involve the development of socio-political technological artefacts. The use of technology in these fields can shape the ways people live, work, and interact in and with their built environment, and can have wide-ranging social, economic, and environmental impacts. However, the implications of technology use in these fields are often not fully understood or appreciated by the public and the very citizens who are affected by these artefacts.
The development of free and open-source tools for AEC and other fields may contribute to raising awareness about the implications of technology use in several ways. First, open-source tools are typically freely available and transparent, allowing citizens to access, use, and modify these tools for their own purposes. This openness can empower citizens to become active agents of technological development, enabling them to understand how technology works, experiment with different design and construction approaches, and contribute to the development of more sustainable, inclusive, and resilient technological artefacts. By providing access to open-source tools, citizens can gain a better understanding of the design and construction process, the choices and trade-offs involved, and the implications of these choices on their communities and the broader society.
Furthermore, the development of free and open-source tools can foster a culture of collaboration, participation, and co-creation among diverse stakeholders, including citizens, practitioners, researchers, and policymakers (Yap, Janssen, and Biljecki 2022). Free and open-source tools are often developed through collaborative and community-driven efforts, involving input from various stakeholders with different perspectives and expertise. This collaborative approach can lead to a more inclusive and participatory technological development process, where citizens and other stakeholders can contribute their local knowledge, needs, and aspirations, and help shape the direction of technological developments. This can promote a sense of ownership and engagement among citizens, empowering them to actively participate in shaping the technological artefacts that affect their lives and communities.
Additionally, the development of open-source tools can promote transparency, accountability, and ethical considerations in the use of technology in AEC and other fields. Open-source tools are typically subject to peer review, community feedback, and public scrutiny, which can help identify and address potential biases, limitations, and ethical concerns in the design and use of technology. This transparency can lead to more responsible and ethical technological development practices, where the implications of technology use are critically examined, and the potential impacts on different social groups and the environment are carefully considered. This could contribute to raising awareness of the implications of technology use by citizens who could potentially become active agents of technological developments in a future smart city scenario.
7.5 Conclusion
This chapter presented and discussed the key findings from Chapters 4, 5, and 6. The research findings of these chapters were organised into the three strategies used to conduct the development of the approach in relation to the achievement of the aim and objectives of this research.
The implications of the systematisation of data-driven urban design processes, the developed integration of a design-data environment, and holistic development of data-driven urban design toolmaking processes were discussed, including their identification during the literature review (Chapter 2), the discussion about their conceptualisation during the findings of the semi-structured interviews (Chapter 4), their development process (Chapter 5), and their validation process (discussed in Chapter 6).
The next and final chapter, 8, presents a summary of the key findings, the contribution of the research, the limitations, and future research directions.
Computational Toolkit Prototype - MEGA-POLIS: https://github.com/victorcalixto/mega-polis↩︎